Energy Transitions Require Innovation in Power System Planning
The relationship between electricity supply and demand changes in low carbon systems
The past year has seen a rising number of countries pledging to reach net zero emissions in the coming decades. Even many countries without net zero pledges are pursuing increasingly ambitious decarbonisation plans. Meeting these targets will reshape the power sector in the coming decades.
In 2021, the IEA released its landmark global roadmap, Net Zero by 2050, which detailed two parallel transformations for the power sector as it decarbonises. The first is that variable renewables will increasingly be the foundation of low carbon power generation. The second is that power will be responsible for an increasing share of total energy demand, as electrification becomes a pathway to decarbonisation. Emerging economies are central to global emissions reduction, since they typically have high shares of fossil fuel generation and because electricity demand is expected to significantly increase along with economic growth and greater electricity access.
Increasing shares of variable renewable energy such as solar and wind will mean power systems need to become more flexible. At the same time, the traditional providers of this flexibility – mainly thermal power plants – will play a smaller role as decarbonisation objectives require their reduced use. Variable renewable generators depend on the availability of the sun and wind, and thus require complementary technologies to ensure that the balance between supply and demand is maintained at all times.
New technologies – in particular batteries and other energy storage, biomass, and thermal plants either with carbon capture and storage or burning clean fuels – will therefore play an increasing role to supply this flexibility. At the same time, growing use of electricity and new demand sources represent a huge opportunity to create a world where flexible demand is at least as important as flexible supply technologies.
Such a system is going to look very different than today’s power sector, and these changes mean we need to alter how we plan for a secure electricity system. Our understanding of demand needs to be much more than just how peak demand evolves, and our understanding of supply needs to be much more than how much dispatchable capacity is installed and what is the risk of an outage during supply peaks. In short, it is not sufficient to look only at the system's ability to stay balanced during selected tight moments.
This article explores how system planning, and in particular assessments of system adequacy, will need to innovate and evolve to allow power systems to keep delivering secure and affordable electricity supply during energy transitions. It draws on modelling of the Chinese power system undertaken by the IEA.
System planning is getting more complex
Shifting away from centralised thermal power plants as the main providers of electricity makes power systems more complex. Multiple services are needed to maintain secure electricity supply. In addition to supplying enough energy, these include meeting peak capacity requirements, keeping the power system stable during short-term disturbances, and having enough flexibility to ramp up and down in response to changes in supply or demand. IEA modelling for China in the Announced Pledges Scenario in 2060 illustrates how the technologies providing these services will be more varied in a net zero world compared with today.
Power system adequacy assessments have traditionally relied on a reserve margin approach, which measures how much spare or ‘reserve’ capacity the system has during the tightest periods, expressed as a percentage of the peak demand. This approach focuses on the peak demand period(s), and requires an assessment of the availability of different technologies – including generators, grid elements, and demand response providers – at times of system stress. System operators can then target a certain margin to ensure the combined generation and grid infrastructure is sufficient. Target reserve margins vary by region.
In a world with high variable renewables and a larger share of controllable demand, this approach hits its limits – in part because it is more complex to assess the contribution of demand response resources to adequacy, and in part because the way they interact with each other and the system cannot be fully captured in a reserve margin calculation.
As a result, countries and regions are increasingly looking to more sophisticated approaches to adequacy assessment, and in particular to probabilistic Monte Carlo assessments, explored in more detail below. Some regions are already undertaking full Monte Carlo assessments, including Australia, Belgium and France, as well as an EU-wide assessment undertaken by ENTSO-E. The methodologies are still evolving and vary between regions, however these studies can indicate areas to focus on for countries that wish to move towards probabilistic assessments. In particular, these approaches are important to consider for emerging economies that are expecting to deploy large shares of renewables in the coming decades.
Probabilistic adequacy assessments allow many uncertainties to be evaluated together
A Monte Carlo assessment simulates the power system under many possible conditions in order to calculate the risk of certain loads not being served, taking into account the uncertainty of different input variables. This uncertainty can include many aspects: the extent and timing of unplanned generation and transmission outages; inter-annual variability in demand and renewables supply based on weather incidents; and deeper structural uncertainties such as longer-term shifts in weather patterns due to climate change and evolving electricity demand.
Key aspects of uncertainty that probabilistic modelling assessments can capture
Open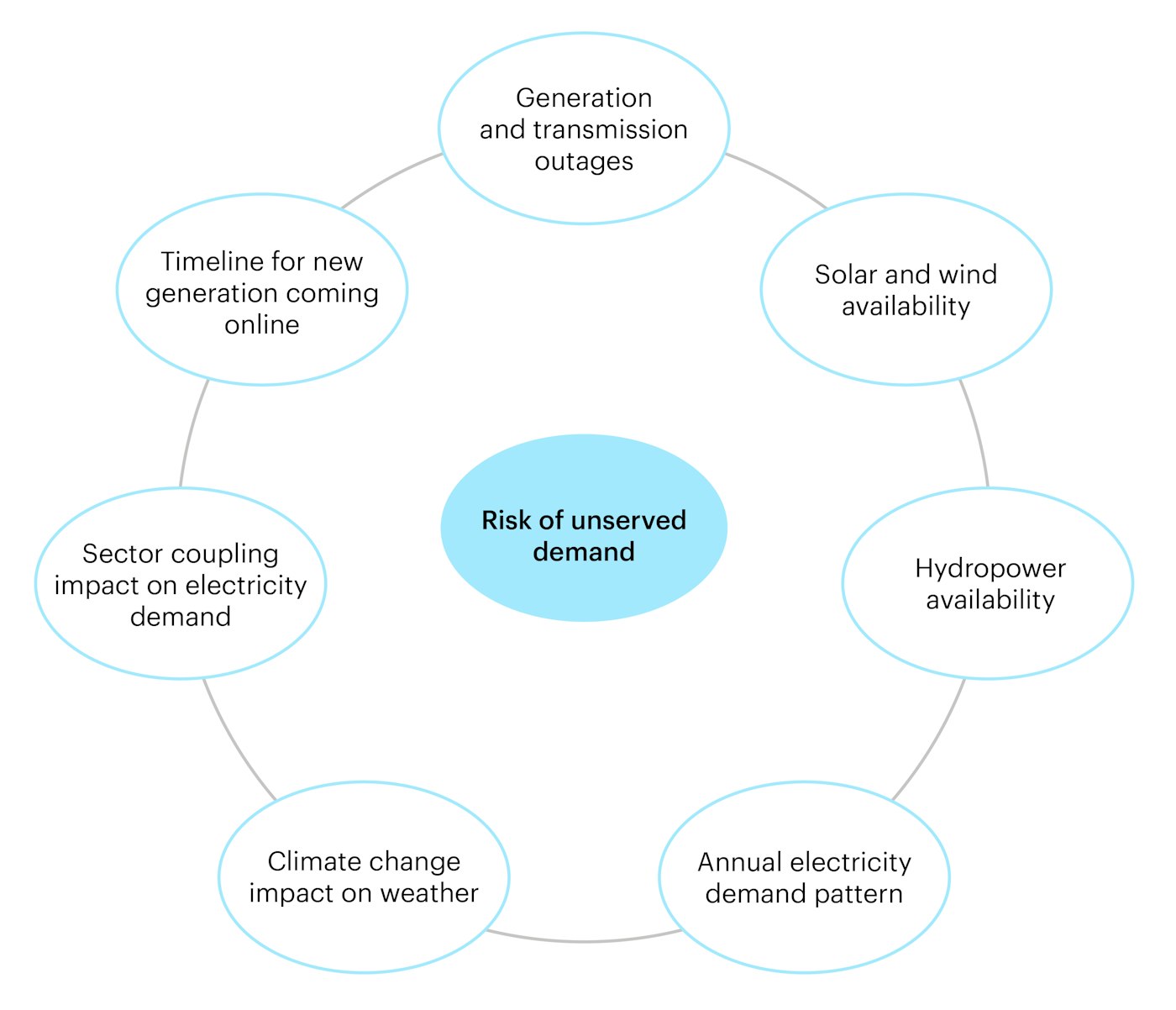
Monte Carlo assessments have many advantages over reserve margin approaches, such as allowing variable renewables to be assessed more accurately in the context of the electricity demand profile. Alongside this, the detailed modelling approach allows sector coupling, including electrification and demand response, to be explicitly represented so that the interactions with renewables availability can be fully captured. Such assessments are also better suited to account for the contributions of interconnected neighbouring regions.
Probabilistic adequacy assessments give more insights for systems with high renewables shares
Monte Carlo assessments derive their statistics from potentially thousands of simulations and express the risk of unserved energy through different reliability metrics. These metrics are diverse, but they can be categorised by two basic parameters. First, whether they assess the amount of load not served (volume), or how often periods with unserved load occur (frequency). Second, whether they focus on the average or ‘expected’ case, or whether they focus on high-impact, low-frequency events.
Due to the simulation approach, these assessments have the potential to give detailed information about adequacy risks, providing information on all different parameters that might affect the acceptability of unserved energy – frequency and magnitude of events, average risk, and what extreme events might look like.
However, today’s assessments do not yet fully realise their potential, in particular because many regions use probabilistic assessments that focus on a single metric. For example, one common metric is the Loss of Load Expectation, which is a frequency-based measure focusing on the average outcome. The Loss of Load Expectation is calculated from a probabilistic assessment of the average number of hours annually where some energy is not served across all simulations. While this is a useful indicator, the Loss of Load Expectation value is insensitive to the size of these events, and as an average indicator does not distinguish between rare but extreme multi-hour events and more frequent, low-impact events.
An adequacy assessment case study undertaken by the IEA for China in 2035 compared two regions under different scenarios with approximately the same value of Loss of Load Expectation. In spite of the similarity in the average statistics, we saw a large gap between the two cases for high-impact, low-frequency events as indicated by the 95th percentile and maximum values for hours of demand not served.
Difference in reliability statistics for extreme events in regions with similar average statistics in China in the Sustainable Development Scenario, 2035
OpenDifference in reliability statistics for extreme events in regions with similar average statistics in China in the Stated Policies Scenario, 2035
OpenThese differentials can have meaningful implications for system planning, as larger events, even if rare, may be less acceptable to consumers than more frequent, low-impact occurrences. Planners should adopt probabilistic approaches, taking into account the frequency, magnitude and duration of unserved energy events, as well as both average and extreme occurrences.
Demand response modelling can build on improved demand forecasting
The rate of demand growth can be one of the largest uncertainties in system planning, and increasing electrification adds to this uncertainty. This is even more challenging in emerging economies, with their faster economic growth and improving electricity access. Better demand forecasting is thus essential for system planning. At the same time, more detailed demand side modelling enables a more sophisticated representation of demand response, in particular because both are limited by data availability.
Demand forecasting often relies on historical profiles and only involves projecting overall and peak growth rates. With the support of detailed sectoral data, including disaggregated sectoral load profiles and specific end uses, this can be refined so the forecast reflects changes in the demand structure over time. The same type of data also allows power system models to represent the flexibility of specific end-use sectors.
Modelling the relationship between electricity demand and temperature is another key area of improvement that is integral to short and long-term demand forecasting, and also provides a foundation for more accurate demand reponses in adequacy models.
Demand response is more complex to represent accurately in probabilistic adequacy assessments than generators. For example, the amount of demand response available varies with the time of day and season since it depends on the consumption patterns of the participating loads, while generators typically have the same maximum capacity at all times of day.
In addition, unlike most generators, demand response providers may only be able to act with limited frequency. For example, demand response contracts between aggregators and their clients may limit the number of activations per month or year, and willingness to participate in these programmes may be based on historical occurrences which are fewer than the contractual maximums.
By fully simulating system dispatch under different conditions, probabilistic assessments have the ability to directly inform aspects such as the frequency and duration of demand response activation in future system conditions.
System planning needs to put all the pieces together
In order to ensure electricity security, in addition to system adequacy, power system planning also needs to consider operational security and resilience. Operational security refers to the ability of the system to maintain or rapidly return to normal operating conditions during and after disturbances. Maintaining operational security requires both system stability – supported by power system inertia and operating reserves – and the flexibility to ramp up and down to maintain the balance between supply and demand.
Components of electricity security (left) and integrated planning aspects (right)
Open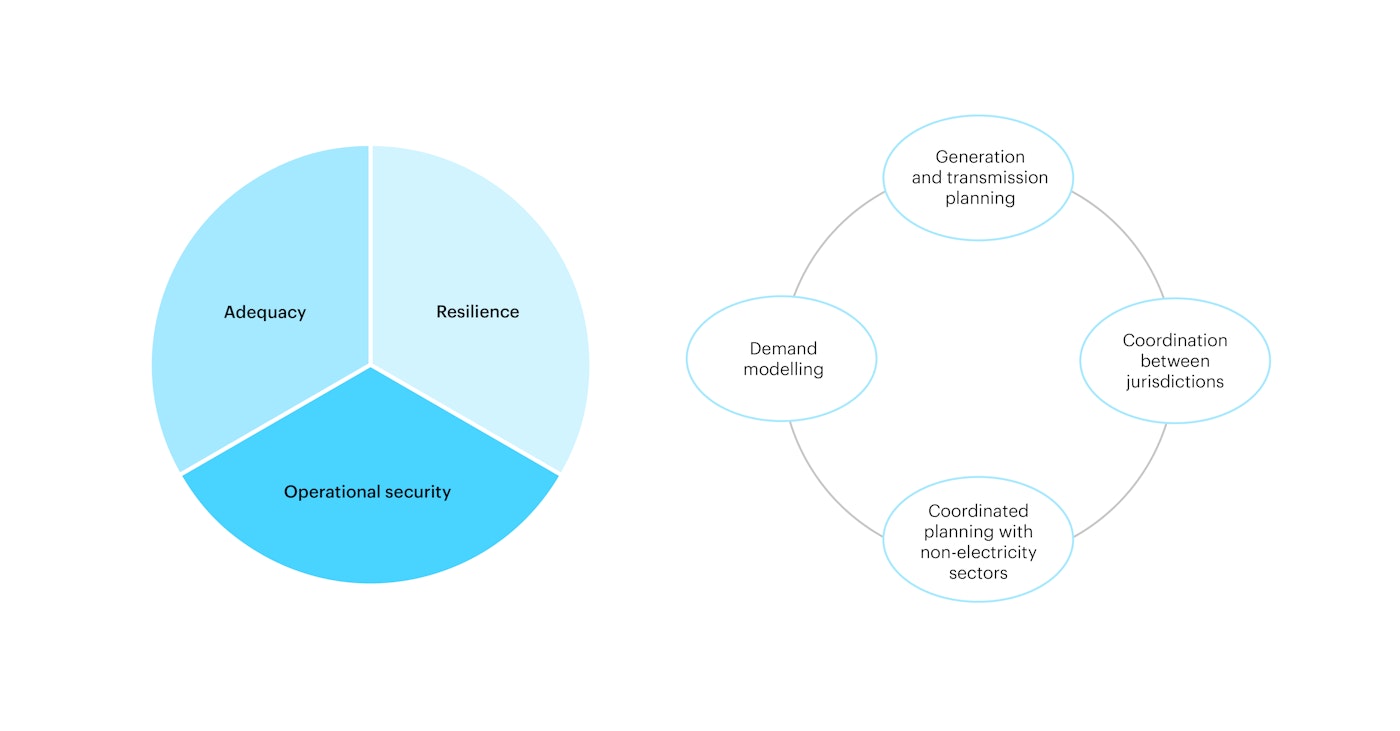
Resilience is the ability of the power system to absorb short- and long-term shocks such as high-impact, low-frequency extreme weather events. With climate change expected to increase the frequency and severity of extreme weather, resilience will be an increasingly important pillar of power system planning.
These three goals are impacted by increased shares of variable renewables. While planning for each involves different modelling approaches, many of the inputs and assumptions are common. Performing studies in a more integrated way creates many opportunities for synergies between different models and for information flow between modelling efforts. For this reason many regions are moving towards increasingly integrated planning across areas that were once treated as silos.
For example, increasing integration of generation and network planning and investment creates opportunities for cost savings by allowing investment decisions to be optimised across the entire system. Interregional planning across different balancing areas also creates opportunities for more efficient investment, accounting for the value that interconnected regions can provide to one another.
In addition, it is also essential to ensure that the prevailing contractual structures allow system operators to utilise the flexibility that the system is technically capable of. Contractual structures that do not allow for operational flexibility can prevent making full use of capable technologies for providing flexibility, for example with rigid long-term fuel purchasing contracts. In addition, integrated planning between the electricity sector and other sectors will become increasingly important as more sectors become electrified and sector coupling increases.
This article has been produced with the financial assistance of the European Union as part of the Clean Energy Transitions in Emerging Economies programme. This article reflects the views of the International Energy Agency (IEA) Secretariat but does not necessarily reflect those of individual IEA member countries or the European Union (EU). Neither the IEA nor the EU make any representation of warranty, express or implied, in respect to the article's content (including its completeness or accuracy) and shall not be responsible for any use of, or reliance on, the publication.
The Clean Energy Transitions in Emerging Economies programme has received funding from the European Union’s Horizon 2020 research and innovation programme under grant agreement No 952363.
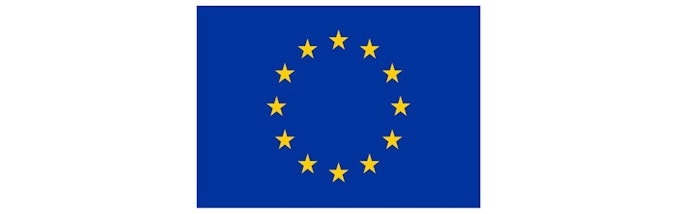